I am looking for some guidance to help me better understand the 68–95–99.7% rule. According to this rule, 68% of the data lies within 1 standard deviation. Now let us apply this rule to a dataset as follows.
5,4,3,2,9,10,4,12,15,20,9,8,3,9,17
Here, the Mean= 8.66 and Std.Dev= 5.45.
So 68% of this data should lie between 14.12 and 3.2 days (+/-1 Std.dev).
Plotted in excel it looks like this:
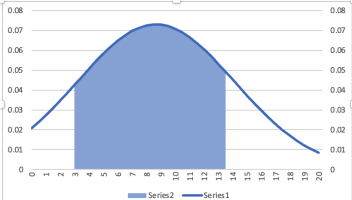
Now according to the dataset, 2 appears once (7%). The range between 3 and 15 appears 12 times (86%). Numbers > 17 appears once (7%).
The range between 3 and 15 (about 1 std.dev) does not lie within 68%, but rather 86%. Hence it does not follow the 68, 95, 99% rule.
Any explanation?
thank you.
5,4,3,2,9,10,4,12,15,20,9,8,3,9,17
Here, the Mean= 8.66 and Std.Dev= 5.45.
So 68% of this data should lie between 14.12 and 3.2 days (+/-1 Std.dev).
Plotted in excel it looks like this:
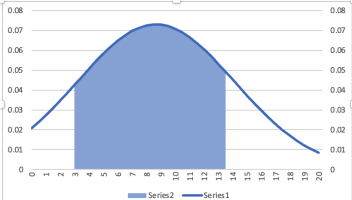
Now according to the dataset, 2 appears once (7%). The range between 3 and 15 appears 12 times (86%). Numbers > 17 appears once (7%).
The range between 3 and 15 (about 1 std.dev) does not lie within 68%, but rather 86%. Hence it does not follow the 68, 95, 99% rule.
Any explanation?
thank you.